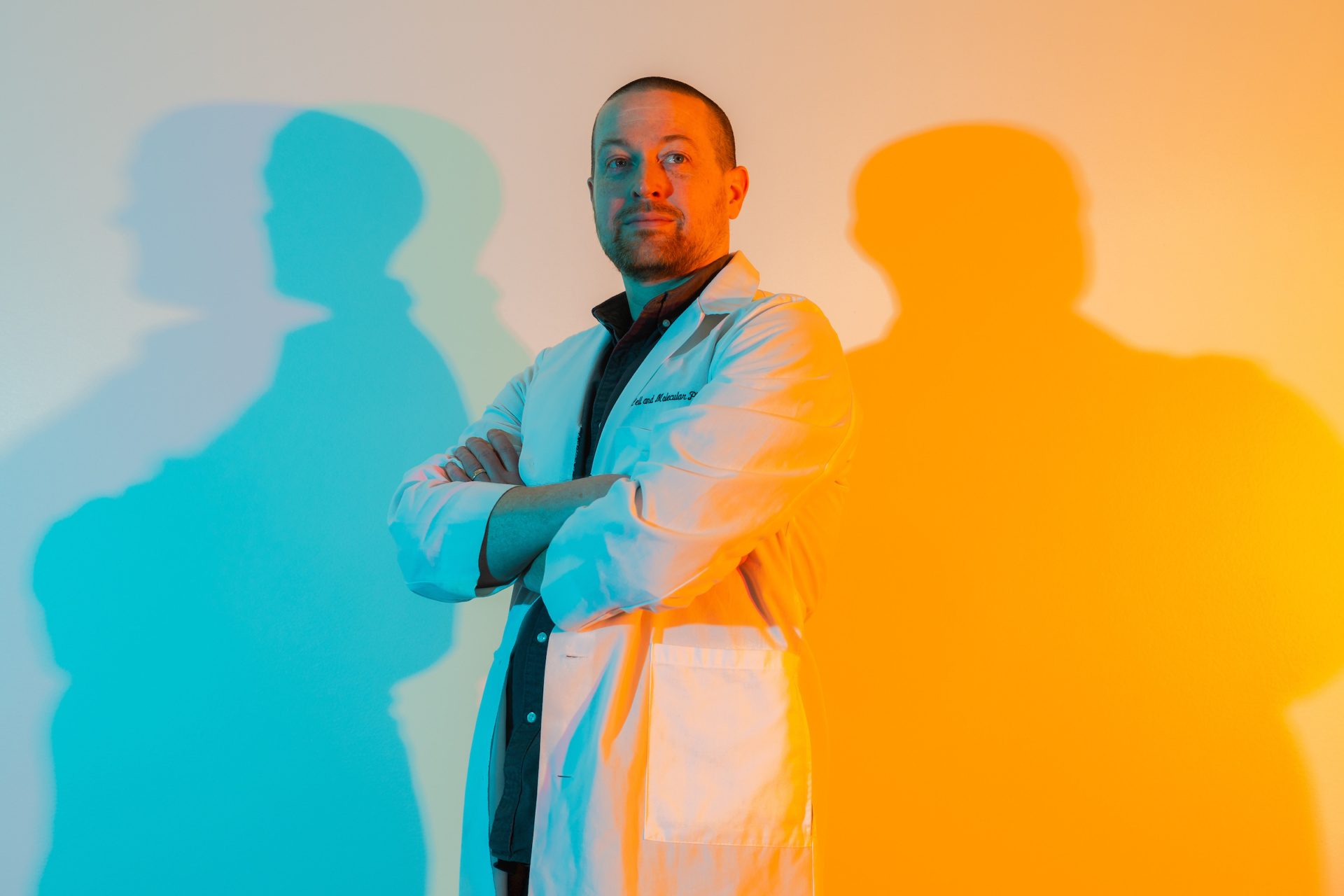
How does a greater understanding of cell movement improve human health?
That’s a question Stritch Associate Professor Patrick Oakes’s lab in the Department of Cell and Molecular Physiology investigates.
Oakes, who earned his PhD in Physics from Brown University, explains that his research focuses on the cytoskeleton, or the “bones” of a cell, and he uses microscopy and machine learning to accelerate and expand this research. His team’s discoveries—understanding cells and developing innovative ways to maximize machine learning—all contribute to understanding cell migration and the physics of living systems with the ultimate goal of helping to minimize disease and improve human health.
Proteins, cells, and movements
Cells contain all the biological tools to make proteins, chemicals, and signals that dictate how living creatures—from the tiniest organisms to plants, animals, and humans—are structured and function. In addition to biochemical interactions, Oakes explained that building complex organisms inherently depends on two key physical processes: adhesion and force generation. In other words, physics. Cells need to hold on to each other to form tissues, and they need to generate forces to change their shape.
Together, these actions allow cells to move, a critical component of processes from cell development to immune response. We see these processes in action every day. For example, when immune cells move from one part of the body to another to mitigate infection, skin cells migrate to close a wound, and skeletal muscle cells change shape as they contract and relax our muscles.
Beyond just generating forces, cells are sensitive to the forces around them, too. Simply changing the stiffness of their local environment—like when we feel lumps in our tissue—can radically alter cellular behavior and fate.
“As scientists, we like to approach research linearly because it’s something we can understand,” says Oakes. “But biology is much more complicated than that.”
Physics, it turns out, is fundamental to understanding and caring for multicellular organisms like humans.
“Bringing together skill sets and knowledge from different disciplines is fundamental to advancing our field and it’s how my lab approaches problem solving. Science is more fun when done together and we all learn something new.”
— Patrick Oakes, associate professor in cell and molecular physiology
Solving a puzzle
“Imagine being given the most complex puzzle you could imagine, with billions of pieces, and no instructions on how to put them together,” says Oakes.
Until Oakes and colleagues from the University of Chicago Department of Physics, along with the University of Chicago’s Institute for Biophysical Dynamics and James Franck Institute, collaborated, no systematic strategy existed anywhere to infer the large scale physical properties of a cell from its molecular components. Without this strategy, it was difficult to learn about various cell processes like adhesion and migration.
To tackle this puzzle problem, the team asked a question: could it use machine learning, a form of artificial intelligence (AI) adept at making connections in overwhelming seas of data, to make predictions to help understand complex processes such as how a cell knows where to adhere and where to pull?
After three years of research, the answer was a resounding yes. Oakes and his collaborators developed a suite of data-driven approaches for biophysical modeling of cellular forces.
Training and leveraging AI
Put simply, AI is a field of study that identifies how to create intelligent systems that can perform tasks requiring intelligence similar to a human’s. AI has several subsets, among them machine learning and neural networks. Machine learning uses data and statistics to develop algorithms to mimic how humans learn; neural networks are a branch of machine learning where the structure is loosely modeled on the brain. Neural networks (or deep learning), use interconnected nodes or neurons stacked in layers, to weight characteristics extracted from images. Both excel at handling, and indeed require, large data sets.
“Microscopy has developed extensively over the previous decades, expanding in both resolution and speed, to produce better images,” says Oakes. “The challenge has been to extract quantitative data from all of these images. The field has really benefited from the approaches brought from other disciplines, such as physics and engineering, and applied to biological systems.”
The team hypothesized that it could train neural networks to predict the forces generated by cells directly from images of a single protein they labeled with a fluorescent marker. Key to this process: Oakes and his team are experts in experimentally measuring these forces and they could compare the predictions from the neural network to their own experimental data.
To start, they chose an adhesion protein called zyxin, which is known to recognize mechanical signals. The results—that images of just this single adhesion protein are sufficient to predict the forces cells generate with over 99 percent accuracy—were astounding.
“Using zyxin was a highly educated guess,” recalls Oakes. “We later tried other proteins associated with contractile processes, but zyxin outperformed them all.”
Buoyed by their success, the team then tested the limits of this approach. Remarkably, the network made excellent predictions even when presented with data it had never seen—including images of other cell types, images taken on other types of microscopes, and even images of cells treated with drugs to alter their function. Once the team demonstrated how broadly one variable (zyxin) could predict how a cell would behave, it could use that model to make other, complex predictions. That discovery is particularly important because it validates using this approach to predict mechanical behavior in cases where proteins can be imaged but where taking actual, physical measurements (such as in tissues) is difficult.
These initial results showed the team that it was possible to distill a cell’s complex processes down to a simpler model. But it left a key question unanswered: How? Neural networks are often referred to as black boxes because the underlying connections they make are opaque and too complex for humans to understand. To overcome this problem, the team set out to determine if a neural network could be interrogated to extract information from it. In other words, could the team actually learn how the neural network was learning?
If the team could deduce how machine learning calculated and drew its conclusions, it could extrapolate and apply it to other problems. The team proposed and showed three different ways to extricate information from machine learning’s black box.
First, they confirmed they could feed it the artificial images where the team had altered individual components, one at a time, to identify the features that the neural network found important. Next, they established they could use machine learning to improve existing models by allowing the network to determine the values of the model parameters. Finally, they demonstrated that it was possible to use an agnostic approach to build a new model from scratch. Together, these findings lay the groundwork for other scientists and labs working with machine learning to understand how AI works.
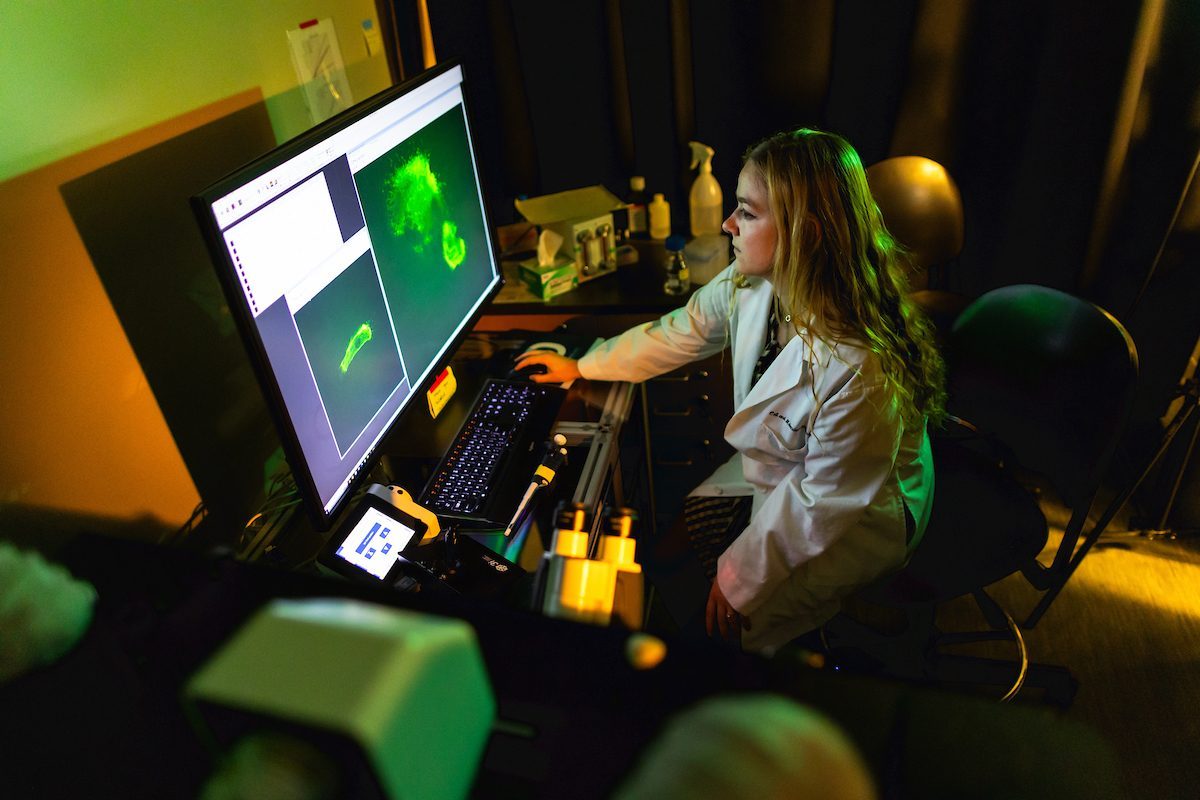
Interdisciplinary collaborators
“Bringing together skill sets and knowledge from different disciplines is fundamental to advancing our field and it’s how my lab approaches problem solving,” says Oakes. “Science is more fun when done together and we all learn something new.”
A postdoctoral scholar in the Oakes lab, Stefano Sala, PhD, performed the vast majority of the experiments for this project, while Matthew Schmitt and Jonathan Cohen, two University of Chicago graduate students, built and implemented the machine learning models. Oakes, along with University of Chicago Professors Margaret Gardel, PhD, and Vincenzo Vitelli, PhD, guided the project. The journal Cell published the research this year.
“We are training scientists to be fluent in the languages of both biology and physics,” says Oakes. That fluency is evident in his lab, an interdisciplinary hub combining physics, biology, engineering, and computer science expertise. It’s where faculty, graduate students, and postdoctoral scholars ask—and answer—fundamental questions about how the human body functions at its most elemental level. Surrounded by screens filled with striking, colorful images of cells that look more like art than science, Oakes and his team are building out the foundational knowledge used to develop new approaches to aiding human health and treating disease.
What question will Oakes ask—and answer—next? “This work was built from images that were single snapshots; linking them together through time is the next big hurdle. The future is never certain,” Oakes says, “but every question leads to more questions, so we’re looking forward to continuing down this path of discovery.”